Configurations for D-optimal Designs
Designed experiments in Cornerstone are often based on D-optimal designs which are flexible enough to handle special situations like “categorical factors” categorical factors, especially those with more than 2 levels
- “constraints” constraints in design space
- “inclusions” include existing (or forced) runs
- “user defined” individual mathematical model edited by the user “number of runs” external restriction for number of runs (e.g.lotsize)
- “block size” arbitrary block size for the blockfactor
The criteria for the design, namely the determinant of the information matrix after rescaling the factors to standard range fits well to the embedding theory and its reciprocal is often called generalized variance. In addition, a maximized determinant minimizes the coefficient confidence intervals. The construction of D-optimal designs relies on a iterative algorithm which uses a list of settings and conditions from the planned experiment under consideration. This report describes how such settings can be chosen in an optimal way to get even better values for the mentioned generalized variance. The results concerning the algorithm are worked into Cornerstone 6.1. However, better settings for the framework parameters in the hand of the experimenter are also investigated to give recommendations in this field.
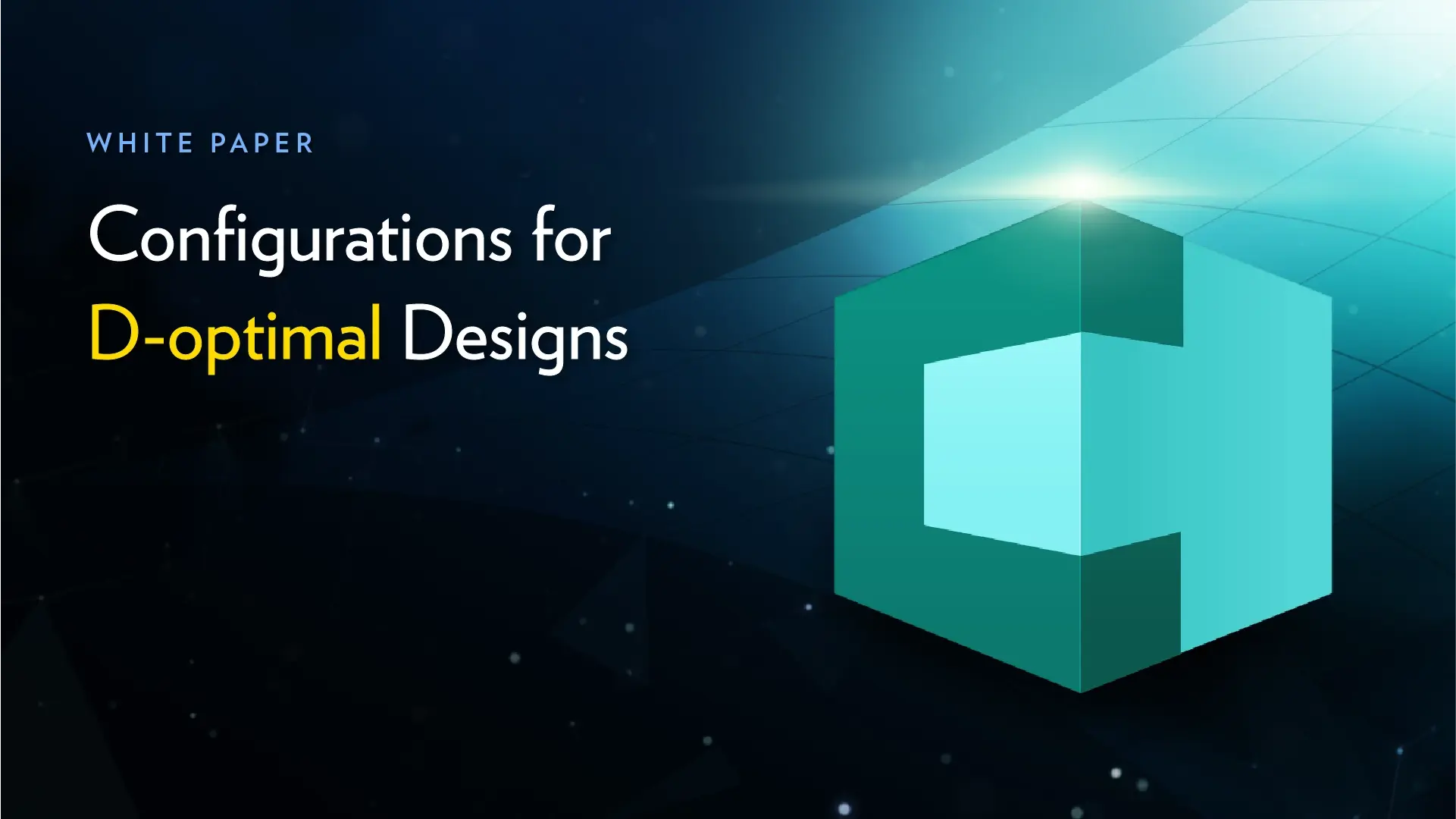
Download Here
Explore More Pages
Discover valuable resources—from our latest news, events, and webinars to white papers—that offer insights into manufacturing automation and innovation.
Let’s Discuss Solutions with camLine's Experts
Our team is ready to deliver tailored solutions that streamline your production, improve product quality, and maximize efficiency across your operations. Tap into camLine’s decades of expertise in digital transformation to overcome your manufacturing challenges.